Matthias has already written his Master's thesis in cooperation with tmc and, after successfully completing his studies this summer, is starting full-time as a programmer at the Linz and Vienna-based employer tmc. In his free time, Matthias regularly seeks out sporting challenges.
Artificial Intelligence – how does it improve track maintenance?
You are most likely already using AI in business and at home. When was the last time you said… Siri? Alexa? or you asked your car to “call mom”? So AI might seem new, but it isn’t. In contrast to natural intelligence, artificial intelligence does not involve consciousness and emotionality. Rather, AI is most often a software running on a device. It perceives its environment and is programmed to take actions that maximize the chance of successfully achieving a set goal.
At tmc, we are using Artificial Intelligence algorithms to give the tamping machine a more human-like perception of tamping. Our Tamping Assistant (tmA²) is a self-learning suggestion system. The track environment is mapped in a digital data representation. Highly specialised algorithms calculate obstacles and other environmental details from the digital data representation. These details are used by the digital tamping assistant to derive actions that are then suggested to the user. This is, in principle, how artificial intelligence in our tamping assistant facilitates operation and ensures the precise restoration of the track position.
Tamping like a pro with Sensor Fusion & AI
We’ve taken tamping to the next level by working with AI-supported laser and light sensor technology. All sensors are read into an environment model using Sensor Fusion. Among other things, point cloud data and camera images are fused. The result is a data-based model of the environment that covers the working area of the machine. The Fully Convolutional Neural Network (FCNN) transforms the sensor information, such as distance information, into a semantically higher-order information space, in our case the track environment information. Subsequently, the reinforcement learning technique is applied to derive actions from this high-level information. Reinforcement learning is a newer paradigm in the field of machine learning. It is primarily used in the field of autonomous driving, but at tmc, we use it for tmA².
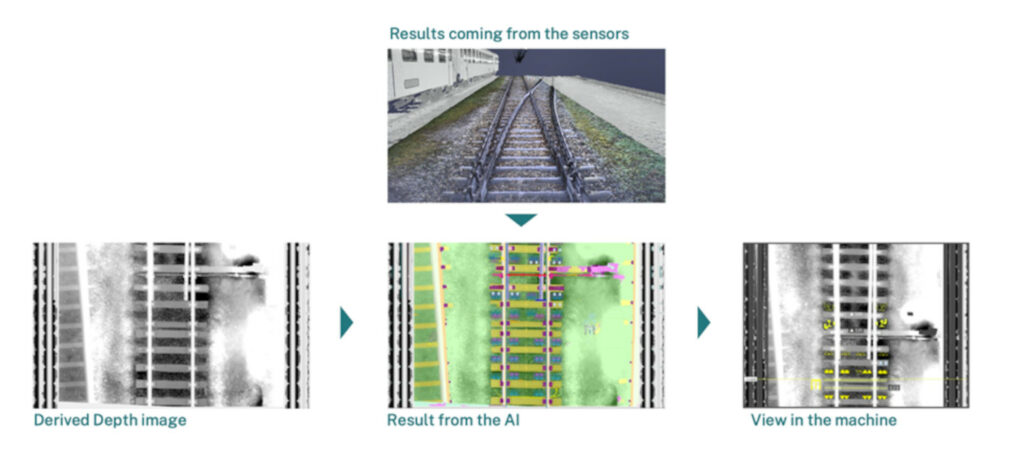
Read more about tmA² to find out about the benefits of tamping with tmA² or contact us for a detailed presentation.